
Written by Scott Wilson
Narrow and general artificial intelligence are two ends of a spectrum of possible capabilities for machine-based intelligence. A narrow AI system may only apply logic and reason to certain specific tasks and functions. A general AI is expected to be able to apply human-level reasoning in any area in which a human might do so.
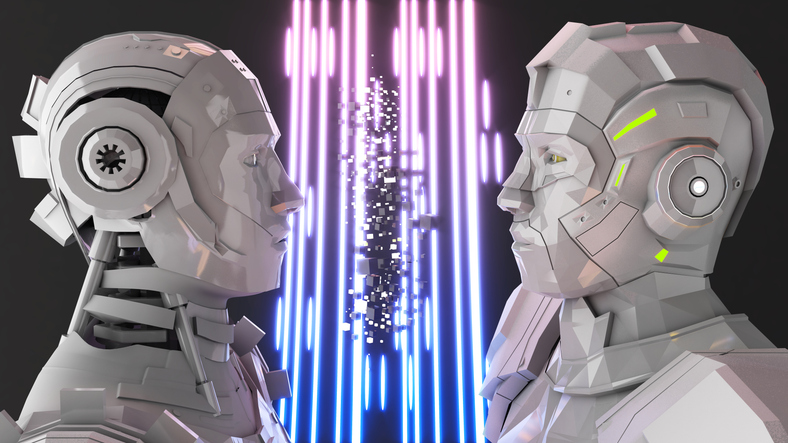
If there is one thing that has become crystal clear in the latest crop of sophisticated artificial intelligence chatbots and analytical systems, it’s that they have some impressive reasoning skills… but not ones that have anywhere near the breadth of human logic.
This is the great divide within the world of artificial intelligence:
- Narrow AI systems that show impressive generative and thinking skills in well-defined tasks
- General AI systems that have a human-like ability to engage those skills in any task
There’s no such thing as a general artificial intelligence yet. But the possibility of such machines may be the motivating force behind the entire AI industry today.
Researchers Stumble When Trying To Draw Lines Between General and Narrow AI
Indeed, one of the major challenges in AI research and development today is simply figuring out where to draw the lines between narrow AI (also known as weak AI) versus general AI (sometimes called strong AI). It ultimately comes back to the nature and definition of intelligence itself.
Definitions of artificial intelligence in general are as fuzzy as the definition of intelligence, so naturally there is also a lot of debate over what exactly constitutes narrow versus general AI.
As much as artificial intelligence is a highly technical field, this is a question that transcends technical answers. Various authorities and researchers have defined it in different ways:
- “…algorithm-based technologies that solve complex tasks by carrying out functions that previously required human thinking.” ~ United Kingdom Information Commissioner’s Office
- “…a machine-based system that is designed to operate with varying levels of autonomy and that can, for explicit or implicit objectives, generate outputs such as predictions, recommendations, or decisions that influence physical or virtual environments.” ~ European Union Artificial Intelligence Act
- “A machine-based system that can, for a given set of human-defined objectives, make predictions, recommendations, or decisions influencing a real or virtual environment.” ~ State of California
- “Engineered system that generates outputs such as content, forecasts, recommendations or decisions for a given set of human-defined objectives.” ~ International Organizations for Standardization
- “…highly autonomous systems that outperform humans at most economically valuable work.” ~ OpenAI
These are, in some cases, already outdated, and sometimes at odds with one another. What they reveal is several different approaches to what constitutes a machine intelligence. They may evaluate it:
- …by the actions or output of the system
- …by the role humans do or do not play in its operation
- …by the actions of the underlying technology it uses
Some of these definitions recognize the differences between strong and weak AI, while others leave the level of capability out of the equation entirely.
Why Does the Difference Between Strong AI and Weak AI Matter?
These definitions matter because a specific intelligence may be judged differently as narrow or general based on process, results, or autonomy. In fact, some people assert that even chatbots are examples of general artificial intelligence due to their independent linguistic capabilities.
Most AI engineers, however, will take a practical, results-oriented approach to evaluating the differences. In fact, for many of them, the performance and skill at a specific set of tasks is all that is really important. Overarching philosophical questions won’t enter into consideration.
But from the perspective of society, there’s a lot riding on the distinction between general and narrow AI. Questions of regulation, bias, fairness, and accountability will all ride on those answers.
It’s also the stated goal of most of the major players in AI right now to achieve general AI. So many of their attempts at building narrow artificial intelligence systems are mostly intended to pay off with bigger and more capable things down the road. So if narrow AI is just a way station on the way to the real deal of AGI, both society and business planners need to understand the differences… and relationships.
Some Similarities Are Found Between General and Narrow AI
Although they are worlds apart in terms of capabilities, there are still many important similarities between narrow and general artificial intelligence.
At a fundamental level, either kind of AI will share some common abilities to enable intelligent actions.
- Perception - AI must have the ability to take in relevant data about the environment. In other words, it must have certain givens to reason with and about.
- Analysis - Within that information, it must have the ability to detect patterns and connections.
- Learning - Finally, it has to be able to use those patterns to develop an understanding of the data which is accurate and actionable—and it must be able to update that understanding over time as it is exposed to additional information. This could be said to be the step that separates AI from conventional computer programs, which are limited by the code they are originally programmed with.
The big differences will be in the fields where they are able to exercise those abilities. A narrow AI will be restricted in some ways as to what it can perceive, learn, and analyze. A general AI should be able to apply those abilities to any area a human being could use them in.
It’s entirely possible for narrow AI to exceed the performance of an AGI system within its own specific area of expertise.
That doesn’t necessarily imply that the AGI would be better than a human, or even than a narrowly focused artificial intelligence.
Looking at the Relationships Between Narrow and General AI
There are schools of thought within the world of AI research that suggest that accelerating and extending current narrow AI abilities may naturally lead into artificial general intelligence. In other words, that by throwing enough text and enough training into a generative pretrained transformer, eventually it will gain broader reasoning skills and more general abilities.
There’s been some evidence for this thinking in testing that appears to show sudden leaps in performance in LLMs. These apparently emergent capabilities come as models are trained on more and more data. At a tipping point, they suddenly demonstrate the skills required to improve their math abilities, solve thorny logic problems, or make connections between concepts that had previously been inaccessible.
Not everyone agrees that these demonstrate actual improvements in reasoning ability. In particular, research in 2023 suggested that the apparent leaps were actually statistical artifacts emerging from how the tests were performed.
One thing that everyone can agree on, however, is that the overall abilities in those models continue to improve over time.
Narrow Artificial Intelligence Looks Smart Only From a Certain Angle
Narrow, or weak, artificial intelligence is by far the easiest level of AI to define. These are demonstrations of applied intelligence in limited functions or at specific kinds of tasks. The examples are all around us:
- Speech recognition and generation
- Facial recognition
- Navigation and route-finding
To develop these skills, machine learning algorithms are typically fed terabytes of data that are specific to the challenge domain. Natural language processing algorithms aren’t trained on mapping data; computer vision systems aren’t handed stacks of Shakespeare to learn from. So it’s not surprising that their abilities are limited to the conclusions that can come from that training material.
Yet narrow AI can even be demonstrably smarter and more capable than humans in these tasks. For example, DeepMind’s AlphaFold was able to outclass generations of human researchers in predicting possible protein structures. Specially trained game-playing AI like Deep Blue and AlphaGO have reliably been able to outplay even the best human competitors in those games.
But it’s telling that you can’t sit AlphaGo down in front of a chessboard and have it replicate the feat. Deep Blue can’t describe how to make a cup of coffee or fold a pile of laundry.
As powerful as narrow AI can be, it can’t be even mediocre at most things.
General Artificial Intelligence Is Intelligent About Everything
A general intelligence, on the other hand, may not excel at any particular application of intelligence, but can apply it to anything it can perceive.
AGI should be able to digest that stack of Shakespeare and learn not just of grammar and rhythm and poetry, but also of betrayal and loss and victory. It should infer the power structures of human society and the passage of time. Fed further English literature, it should find the echoes of those themes through the ages, and trace their impact in art and history.
And it should be able to take that knowledge of the world and apply it in other tasks. It might paint a picture of the monster Caliban or compare a modern political figure to the character of Macbeth.
You can see how such abilities quickly blossom into everything we expect people can do. They can ask questions; imagine alternatives; build tools; do science. It wouldn’t rely entirely on humans for its training material. It would generate such material itself.
All of this requires one major leap that narrow AI is missing: a world model that information and logical relationships can be plugged into.
The Hidden Mystery Behind General Intelligence
When you look at human, or natural, intelligence, though, a model doesn’t seem like quite enough. For thousands of years scholars and philosophers have tried to figure out what exactly it is that makes human intelligence special. There is a drive behind questions and uses of the imagination. Where would that come from in a machine?
Mostly what they come up with is consciousness. But consciousness is even harder to define than intelligence. Many philosophers and scientists aren’t even convinced it exists.
Measuring AGI calls the very nature of intelligence itself into question.
A lot of the discussion around AGI gets hung up on self-awareness.
It’s not clear that an intelligent machine needs to be conscious of itself in the way that human intelligence is. But many cognitive scientists do believe it needs to be something more than just a disembodied collection of algorithms hovering in an electronic void. Psychologists have repeatedly tied intelligence to the physical and emotional experiences that humans process.
And if you define intelligence as a measure of fitness for the environment, then it seems that any AGI will need the kinds of deep and ongoing sensory interaction with that environment that we have.
While an AGI without consciousness may apply logic and reasoning skills on par with a human, there’s every reason to believe that it would be very unlike humans in behavior and thought processes. And that’s a both exciting and frightening idea.
Artificial General Intelligence Raises Both Hope and Fear in AI Researchers
There is a lot of fear and hype surrounding the idea of artificial general intelligence.
The hype comes from an apparently limitless and inexpensive resource for society to draw on to perform almost any task that people perform today. From driving garbage trucks to filing TPS reports, fans of AGI see a future where people exist in happiness and prosperity with all the worst requirements of work and production taken care of by machines.
The fear comes from a technical argument about the speed and upgradability of digital systems. With processors that work at many times the speed of the human brain, the argument goes, any AI that achieves general intelligence will very quickly be able to accelerate itself to super-intelligence.
The outcomes for humanity living side-by-side with a kind of intelligence we cannot rival and may not even be able to understand are uncertain at best.
Both of these positions suffer from a lack of information. On the hype side, it’s far from obvious that the economics of AGI will result in universal peace and happiness. Robot warriors and servants for the very rich may be the actual outcome.
On the fear side, it’s unclear that general reasoning skill will automatically offer a path to greater intelligence… after all, it’s not clear such systems would be motivated to pursue such outcomes. Even if they were, there’s every chance the complexity of their operation wouldn’t make that an easy feat. Humans, after all, can’t upgrade our brains just by thinking about it.
The positions advocating faster AI development versus slower development, respectively, are known as boomers and doomers.
As always, it’s largely the unknown that drives fear of AGI. As many modern AI researchers and theorists have pointed out, however, the real-world impacts of narrow AI are both much clearer and potentially just as devastating in their own way:
- Deeply encoded bias against minorities
- Widespread job losses creating mass unemployment
- Further concentration of wealth and power in the hands of the few
Regardless of whether they come from advances in narrow or general artificial intelligence, real human impacts will be felt around the world.
Exploring Hard Questions in Any Kind of AI Requires Advanced Degrees
Everything you’ve read here just now is the tiniest tip of the iceberg in questions of narrow versus general AI. To truly get a grip on the questions themselves, let alone taking a stab at the answer, you’ll need to join the ranks of deep thinkers who have gone as far as they can in studies of computation, statistical theory, data, and the inner workings of the mind: earn a PhD in Artificial Intelligence.
Such programs allow students to ask big questions and pursue the research projects to answer them. Half or more of the five years you can expect to spend in a PhD program are likely to be devoted to exploring your doctoral dissertation topic. Building evidence, working with other scientists, and exploring the logical deductions of your experiments is how AI research will develop the answers to the questions of narrow versus general AI.
What Kind of AI Will You Build?
Graduates with those PhD programs will do far more than just answer grand philosophical questions about the nature of machine intelligence. They will also be the people who design and build those intelligences… at least, until the machines can finally build themselves.
So many of the choices in how and what sort of artificial intelligence will come to share our future will be up to people like you. What will you choose?
One thing you can’t do is sit on the sidelines. The 2023 Expert Survey on Progress in AI, an annual survey of nearly 3,000 experts in the field, gives a 50 percent probability of achieving human-level machine intelligence by 2047. That’s more than a decade sooner than the results of the 2022 survey.
The moat between narrow and general AI is shrinking fast. Be ready when it closes!